The goal of Lu Group’s research is to model and simulate physical and biological systems at different scales by integrating modeling, simulation, and machine learning, and to provide strategies for system learning, prediction, optimization, and decision making in real time. Our current research interest lies in scientific machine learning (SciML) and artificial intelligence for science (AI4Science), including theory, algorithms, software, and its applications to engineering, physical, and biological problems. Our broad research interests focus on multiscale modeling and high performance computing for physical and biological systems.
Ph.D., Master’s, & Undergraduate Students and Postdoc Opening: We are looking for Ph.D., Master’s, and undergraduate students, and Postdocs to work on scientific machine learning (SciML) and artificial intelligence for science (AI4Science). Students in statistics, mathematics, physics, computer science, engineering, or related majors with proficient coding skills are welcome to apply. Please feel free to contact me with CV (and/or transcripts, sample publications) attached if you are interested. For more information, please check the Ph.D. programs in Statistics and Data Science and Applied Mathematics, and postdoctoral positions in Data Science (Job posting), Applied Mathematics, and Neuroscience.
Recent News
- DeepXDE is the Most Read article in SIAM Review. (Feb. 23, 2024)

- New paper on arXiv: Identifying heterogeneous micromechanical properties of biological tissues via physics-informed neural networks. (Feb. 16, 2024)
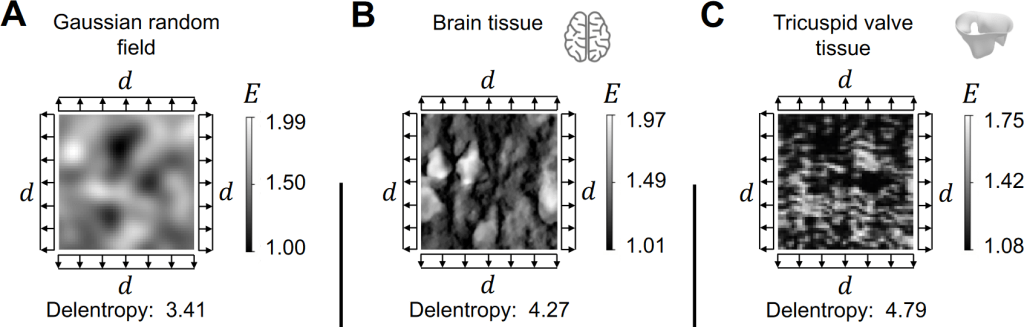
- New paper on arXiv: Speeding up and reducing memory usage for scientific machine learning via mixed precision. (Jan. 31, 2024)
- Lu Lu gave a talk on “Accurate, efficient, and reliable learning of deep neural operators” at Stanford University, Department of Mechanical Engineering, Mechanics and Computation Seminar. (Dec. 7, 2023)
- NVIDIA Deep Learning Institute has launched the Science and Engineering Teaching Kit developed by George Karniadakis, Raj Shukla, and Lu Lu in collaboration with NVIDIA. This course is available for free download for universities from the NVIDIA websites (here and here). For more information, please check out the news. (Nov. 13, 2023)
- Highlight on AAS Nova: DeepONet offers an easier, faster way to model planet-forming disks. See the paper in Astrophysical Journal Letters. (Oct. 9, 2023)
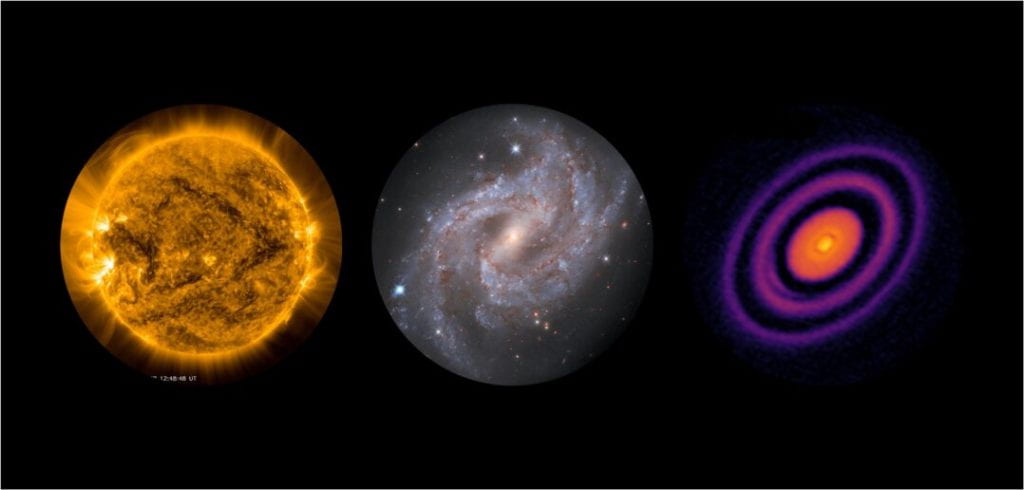
- New paper on Computer Methods in Applied Mechanics and Engineering: Fourier-DeepONet: Fourier-enhanced deep operator networks for full waveform inversion with improved accuracy, generalizability, and robustness. (Aug. 11, 2023)
- New paper on Applied Mathematics and Mechanics: Effective data sampling strategies and boundary condition constraints of physics-informed neural networks for identifying material properties in solid mechanics. (July 3, 2023)
- New paper on arXiv: Deep learning for solving and estimating dynamic macro-finance models. (May 18, 2023)
- Congratulations to Anran Jiao on winning the Master’s Outstanding Academic Award of Penn Engineering. (May 11, 2023)
- New paper on Computer Methods in Applied Mechanics and Engineering: Reliable extrapolation of deep neural operators informed by physics or sparse observations. (May 2, 2023)
- New book chapter on Computational Modeling of Signaling Networks: Systems biology: Identifiability analysis and parameter identification via systems-biology-informed neural networks. (Apr. 20, 2023)
- New paper on arXiv: Fourier-MIONet: Fourier-enhanced multiple-input neural operators for multiphase modeling of geological carbon sequestration. (Mar. 8, 2023)
- Congratulations to Benjamin Fan and Edward Qiao on winning the Honorable Mention (Economics and Financial Modeling) of 2022 S.-T. Yau High School Science Award USA. (Nov. 21, 2022)
- Lu Lu gave a plenary talk on “Learning operators using deep neural networks for multiphysic, multiscale, and multifidelity problems” at Mathematical and Scientific Machine Learning (MSML). Watch the talk on YouTube. (Aug. 15, 2022)
- Congratulations to Lu Lu on winning the DOE Early Career Award. (June 7, 2022)
- Congratulations to Jeremy Yu on winning the Bronze Medal (Computer Science) of 2021 S.-T. Yau High School Science Award USA. (Nov. 16, 2021)